Our research themes revolve around the integrated application of remote sensing, numerical modeling, and innovative ground-based observations with the goal of improving fundamental understanding and prediction of seasonal snowpack states and processes.![]() Figure: Sturm (2015) after Raleigh (2013). |
Theme 1: Integrative approaches for mapping mountain snowpack
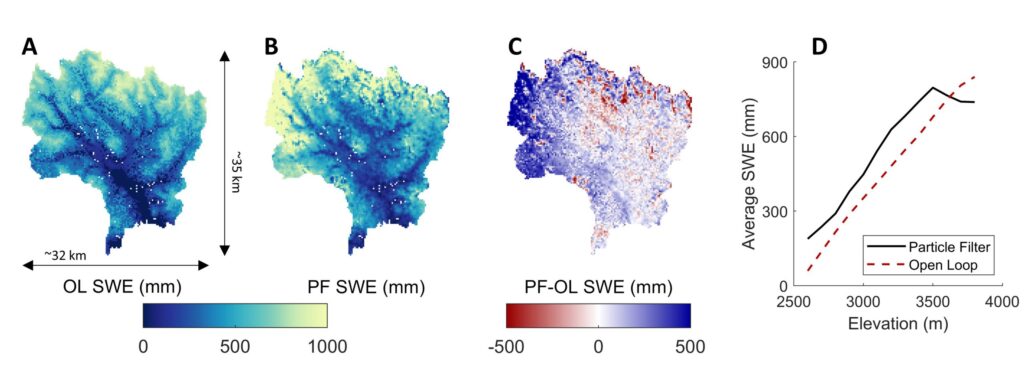
Seasonal snowpack is important to water supply in many watersheds globally. The amount of water in snow – i.e., snow water equivalent (SWE) – can vary significantly in space and time. Ground-based networks are typically too sparse to characterize SWE volume over large scales. Our research examines how we can combine quantitative information from remote sensing with physics-based numerical models to improve estimates of SWE across watersheds and mountain ranges. Our work has focused on data assimilation techniques and SWE reconstruction, with emerging work in machine learning applications to combine process understanding with big data analytics.
Funding: NASA SnowEx / Terrestrial Hydrology Program
——————
Theme 2: Remote sensing of snow cover and surface characteristics
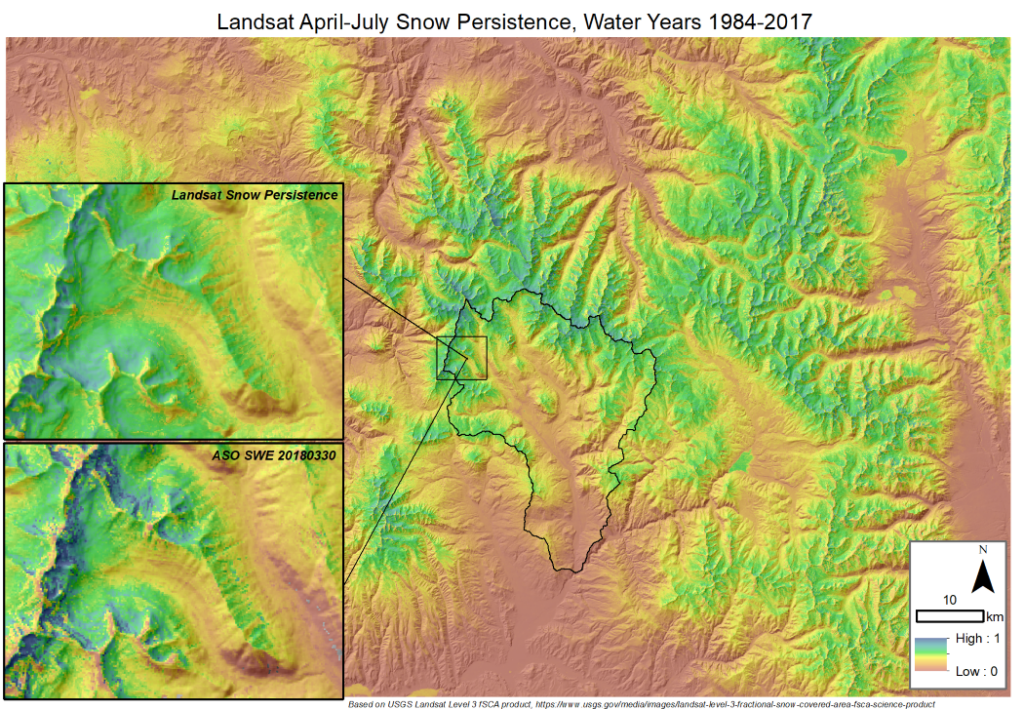
With a five decade record of Earth observations, satellite remote sensing is key for understanding surface processes and change globally. With satellite remote sensing, we can map seasonal snow cover and other snow surface properties. However, long-standing challenges remain. We have gathered on-the-ground datasets of snow cover that have permitted testing of and corrections to satellite remote sensing in its “blind spots” like forested areas. Our research has embraced techniques for quantifying fractional snow cover within pixels and downscaling to ecologically-relevant scales. Our team also contributes to Snow Today, a project that provides near-real-time mapping of daily fractional snow cover over the Western United States with monthly narratives that provide context from hydrologic and climate perspectives. Our emerging work is linking time-integrated metrics of snow presence from satellite remote sensing to SWE distributions..
Funding: NASA, Colorado Water Conservation Board
——————
Theme 3: Snow interactions with forests and climate
In western North America, nearly half of the snow zone is in forests. Yet, routine snowpack measurements have been predominantly made in non-forested areas or clearings that do not represent snow conditions in forests. Forest canopy controls how much snow accumulates on the ground, snowmelt rates and timing, and the ecologically-significant date of snow disappearance. Snow models do not consistently represent these processes, while many types of snow remote sensing are challenged in characterizing forest snowpack. We have contributed novel investigations of snow states and processes in forests, such as: quantifying canopy snow mass based on changes in tree sway, identifying forest-boundary effects on SWE with ground-penetrating radar, and characterizing patterns of snow disappearance in burned and unburned forests with networks of small temperature sensors. We are actively researching how forest canopies influence snow densification processes in continental and maritime climates.
Check out our near-real time data streams from our paired open-forest weather stations in Crested Butte, Colorado:
Forest Site ——- Open Site
Funding: NSF Hydrological Sciences
——————
Theme 4: Physics-based snow modeling, scale, and process understanding
Physics-based snow models encode our knowledge of how snowpacks develop, change over time and space, and melt. Models are a critical tool for integrating disparate data streams (like remote sensing and ground-observations) and are useful for predicting how changing conditions (e.g., changes in forests or climate) will influence snowpack dynamics. While the physics behind many snow-related processes are generally well understood, our predictive capacity with models is hindered by high uncertainties in surface conditions (e.g., local meteorology) and parameters that describe landscape characteristics and processes. Our research has investigated the relative hierarchy of inputs that control uncertainties in SWE modeled with physics-based models. Our emerging work is exploring how process representation and model scale influence prediction of SWE patterns across watersheds.
Funding: DOE, NASA, NSF
——————